Lucia Cipolina Kun
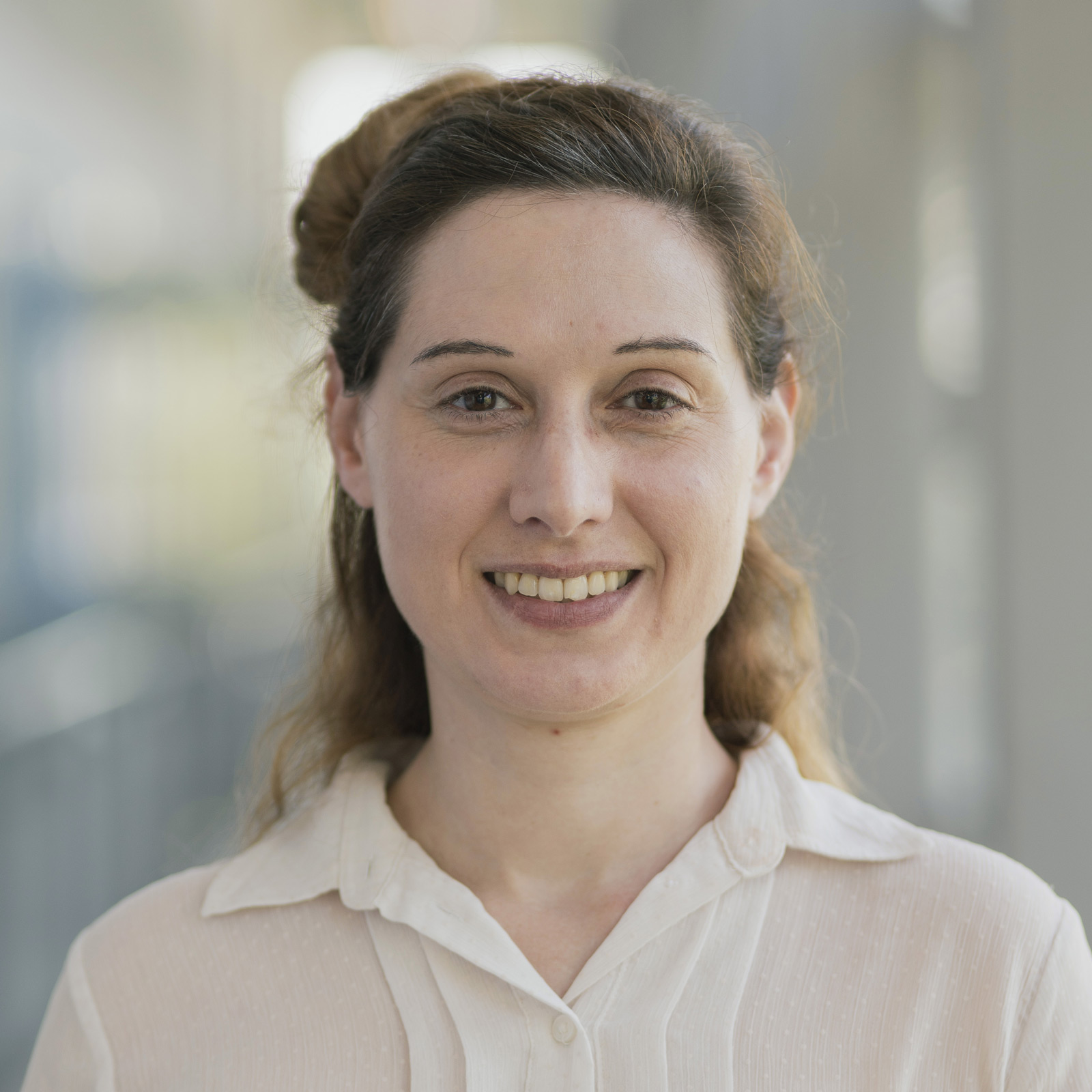
I am a researcher in Artificial Intelligence with a particular interest in Mathematics. My current research combines deep reinforcement learning, multi-agent systems, and game theory. Currently, I am focusing on post-training evaluation and planning for large language models (LLMs). My work aims to design methods for systematically evaluating LLMs in multi-agent scenarios, such as cooperative or competitive games, to uncover their strategic decision-making capabilities and behavioral consistency. This research is novel because it bridges theoretical insights from game theory with practical advancements in LLMs, enabling a deeper understanding of their performance in dynamic, interactive environments. It is particularly interesting as it sheds light on how LLMs can adapt to and influence complex systems, paving the way for their deployment in real-world decision-making tasks requiring collaboration, negotiation, or competition.
My professional background includes roles as a Student Researcher at Google DeepMind, where I developed novel techniques for multi-agent decision processes, and as a Research Intern at Juelich Supercomputing Center, focusing on high-performance computing, large-scale generative models and LLM evaluation. I have also interned at JPMorgan AI Research optimizing reinforcement learning algorithms for trading, and at Microsoft AI, working on model-based offline reinforcement learning for Bing’s search and revenue management. Earlier in my career, I worked as a front office quant at Morgan Stanley, Bank of America and HSBC in NY, implementing machine learning and diffusion models in C++.
I am currently pursuing a PhD in Electrical Engineering at the University of Bristol (expected Feb 2025), focusing on deep reinforcement learning and multi-agent systems. I hold an MSc in Mathematics from the Courant Institute at the New York University, where I specialized in stochastic differential equations.
I am also involved in a side project exploring AI-based art restoration, where I investigate how machine learning can help preserve and restore cultural heritage.
news
Dec 15, 2024 | Our paper on LLM reasoning Alice in Wonderland: Simple Tasks Reveal Severe Generalization and Basic Reasoning Deficits in State-Of-the-Art Large Language Models has been accepted at NeurIPS 2024 Workshop on Scientific Methods for Understanding Deep Learning. |
---|---|
Jul 15, 2024 | I have started an internship at Google DeepMind in the Game Theory and Agents team. My work will focus on multi-agent coordination. |
Nov 07, 2023 | Best student poster award at the First Multimodal AI Workshop in the UK |
May 23, 2023 | I have started an internship at the Tate Britain, where I applied diffusion models to a virtual restoration of the museum’s pieces. The work was part of the Tate’s collection first rehang in ten years. |
Sep 16, 2022 | Best student poster award at the Mediterranean Machine Learning Summer School |
selected publications
- Alice in Wonderland: Simple Tasks Showing Complete Reasoning Breakdown in State-Of-the-Art Large Language ModelsScience of DL workshop. Neurips, 2024